Ray
Posted: Updated:
Keeping track of publicly available technology is an important part of honest machine learning consulting. Almost always, the best solution for a client is not for the consultant to build from scratch. In fact, an informed client should be suspicious of such a recommendation. Primarily, the consultant’s role is to build software connecting powerful tools to the specific operations of the client1.
So we pay close attention to the AI/ML landscape.
RayPermalink
A growing tool is Ray, the AI computing framework. It’s finding its place among leading companies in AI/ML applications:
- Uber used it for strong market optimization improvements and a boost to developer productivity.
- Instacart used it to improve resource utilization, developer productivity and reduced execution time.
- Pinterest used it to see similar gains.
This inspired us to get familiar with RAY, survey the positive and negative2 use cases, and clean up our understanding into a shareable one pager:
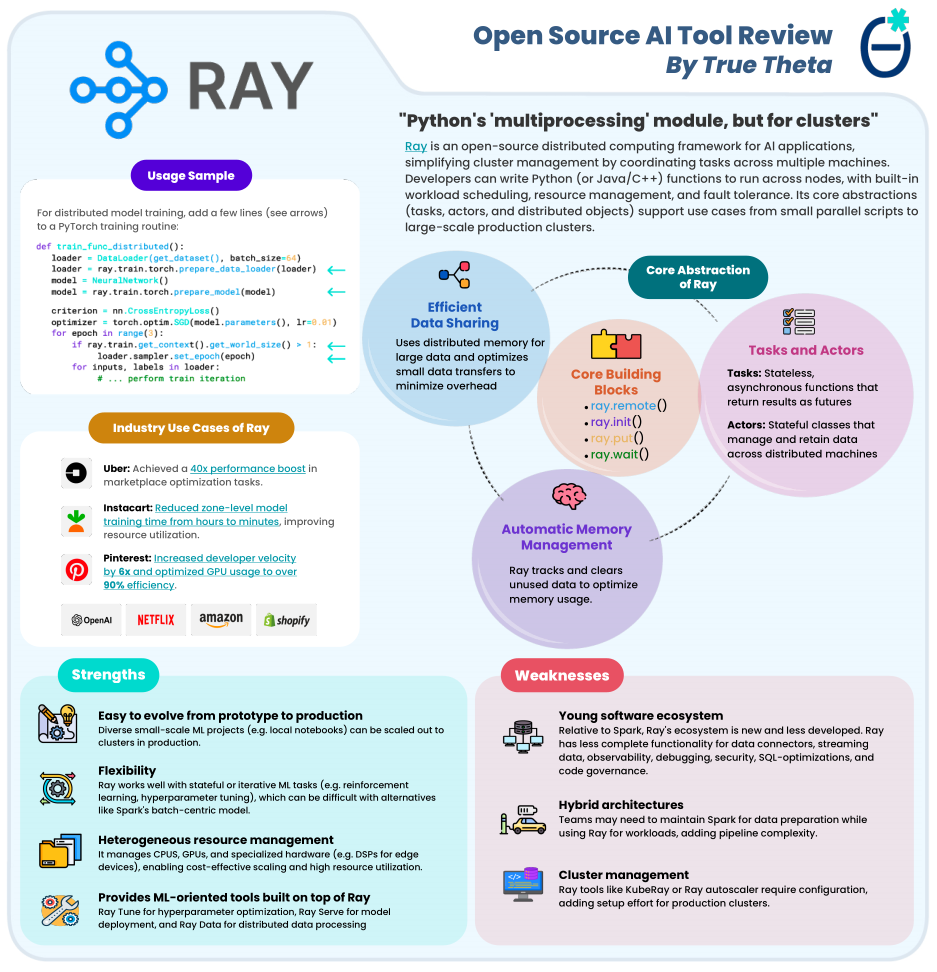
FootnotesPermalink
-
In fact, this makes for no shortage of work. We often observe clients’ asks to be so specialized, no publicly available, designed-for-growth product quite does the trick. ↩
-
Few publicly express what failed, so we rely on our own experience, word of mouth and public discussions (X, reddit and GitHub issues mostly). ↩